Computational Immediacy
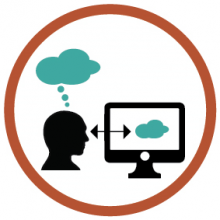
Design intentions are, to a large extent, based on architectural and visual knowledge and subjective aesthetic preferences. Often designers cannot explicitly state these criteria, therefore they use reference images or objects to articulate initial concepts. The translation of rather vague design ideas into geometry is a time-consuming process of trial and error and requires workarounds or mixed media approaches. Concept models are an essential medium for exploring and communicating initial design ideas. They prevent a too early fixation on a “solution” through their purposeful vagueness. Existing modelling frameworks require the designer to choose a geometrical carrier (e.g., NURBS modelling) for a concept model, which already pre-determines the output. The understanding of design as a creative and open-ended exploration requires alternative computational methods that facilitate implicit modelling and imagination within open design spaces.
We will develop a design method for implicit modelling of 3D point clouds through the input of 2D images or sample 3D objects based on transfer learning. The aim is to achieve an immediate translation of specific features retrieved from 3D objects to a model without geometrical predetermination. As compared to traditional machine-learning approaches, transfer learning allows reusing existing knowledge contained in already established learning architectures without fully repeating the expensive training process. Consequently, we will use pre-trained deep-learning architectures. To avoid restrictions by specific meshes or renderings, we will deploy 3D point clouds as a design system. Point clouds have many advantages over meshes in terms of representation of vagueness (e.g., of objects that do not have sharp boundaries but transition zones). Moreover, effective pre-trained networks like PointNET and PointNet++ just recently got available. These enable an effective analysis of topological features and their underlying metrics (distance between sets of points). We aim for a synergetic coupling of implicit modeling based on encapsulated design knowledge in 3D objects and strategic control of models through topological data analysis.